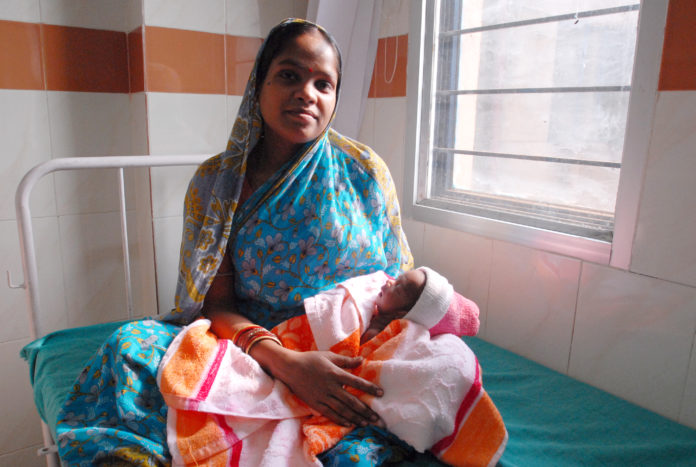
In a study funded by the National Institutes of Research, Israeli and US scientists have developed a model that can use AI to predict PTSD in new mothers based on some statements
New Delhi: An artificial intelligence (AI) programme helped researchers identify potential patients of Post Traumatic Stress Disorder (PTSD) in new mothers. They analysed short statements by these women using AI to predict who was the most likely to develop PTSD.
Worldwide, child birth related PTSD (CB-PTSD) affects about 8 million people who give birth each year, and current practice for diagnosing CB-PTSD requires a physician evaluation, which is time-consuming and costly. An effective screening method has the potential to rapidly and inexpensively identify large numbers of postpartum patients who could benefit from diagnosis and treatment. Untreated CB-PTSD may interfere with breastfeeding, bonding with the infant and the desire for a future pregnancy. It also may worsen maternal depression, which can lead to suicidal thoughts and behaviors.
Investigators administered the CB-PTSD Checklist, which is a questionnaire designed to screen for the disorder, to 1,295 postpartum people. Participants also provided short narratives of approximately 30 words about their childbirth experience. Researchers then trained an AI model to analyze a subset of narratives from patients who also tested high for CB-PTSD symptoms on the questionnaire. Next, the model was used to analyze a different subset of narratives for evidence of CB-PTSD. Overall, the model correctly identified the narratives of participants who were likely to have CB-PTSD because they scored high on the questionnaire. The study was funded by the National Institutes of Health.
The authors believe their work could eventually make the diagnosis of childbirth post-traumatic stress disorder more accessible, providing a means to compensate for past socioeconomic, racial, and ethnic disparities.